Chapter 9 - Digital Divides and Tech Ethics
- pranavajoshi8
- Mar 3
- 14 min read
Updated: Mar 6
Introduction: The Promise and Peril of Uneven Technological
Progress
Throughout our exploration of the AI revolution, we've examined its transformative potential across industries, workplaces, and even our search for meaning. Yet underlying these possibilities is a crucial question: Who benefits from these advancements, and who gets left behind?
As artificial intelligence systems grow more powerful and integrated into every aspect of society, we face a critical inflection point. Technology has always created both opportunities and disparities, but the scale, speed, and significance of AI amplifies these patterns dramatically. The decisions we make today about how AI is developed, deployed, regulated, and distributed will shape power dynamics in our society for generations to come.
This episode examines the emerging digital divides in the AI era and explores ethical frameworks to guide responsible innovation. We'll see how access to AI tools is creating new forms of inequality, how biases can become encoded in seemingly neutral systems, and how different stakeholders are working to build more inclusive and ethical technological futures.
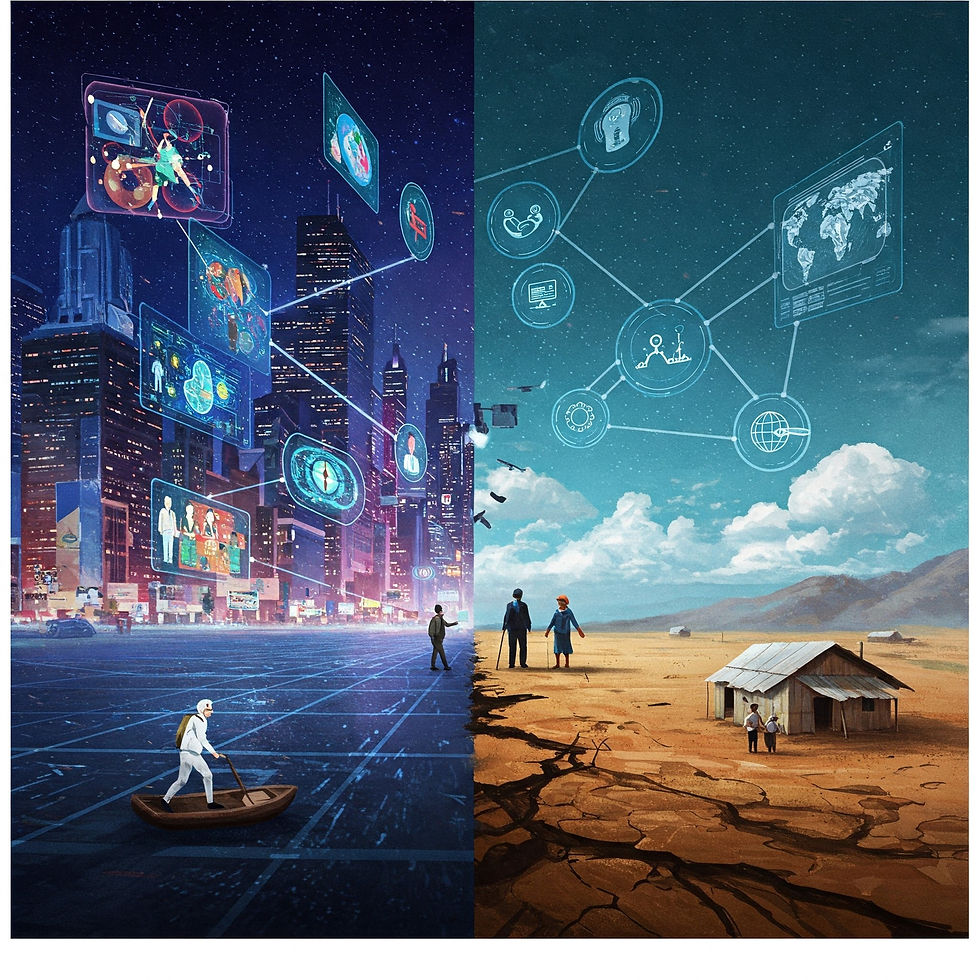
The New Digital Divides
The original "digital divide" described the gap between those with and without internet access. Today's AI-driven divides are more complex and multidimensional, as illustrated in our interactive visualization of these interconnected disparities:
Geographic Divides
AI development is concentrated in specific global hubs—primarily the United States and China, with secondary centers in the UK, Canada, Israel, and parts of Europe. Countries without strong AI ecosystems risk becoming technological dependencies rather than innovators.
Research from Stanford University's Institute for Human-Centered Artificial Intelligence (HAI) confirms this concentration: their 2023 AI Index Report shows that over 70% of global private investment in AI flows to companies based in the United States and China [1]. Similarly, MIT's Technology Review has documented how this geographic concentration creates "AI colonies and empires," with most countries becoming consumers rather than producers of AI technology [2].
Even within nations, rural areas often lack the high-speed connectivity, specialized talent pools, and investment capital needed for AI development. Professor Meredith Whittaker of NYU describes this as "technological redlining," where certain communities are systematically excluded from technological infrastructure and opportunity [3].
Economic Divides
The economic benefits of AI flow primarily to three groups: technology companies developing AI systems, knowledge-economy businesses implementing these tools, and highly educated workers with the skills to develop or work alongside AI. Meanwhile, millions of workers face displacement from routine jobs without clear pathways to new roles.
Research from the Wharton School of Business indicates that AI adoption is creating a "winner-take-most" dynamic in which companies that effectively implement AI gain market share at the expense of slower adopters, potentially increasing industry concentration [4]. This pattern threatens to accelerate existing inequality—as UC Berkeley economist Laura Tyson notes, "The distribution of AI's benefits may mirror and potentially amplify the broader inequalities in our economy" [5].
Skills and Education Divides
A new form of literacy is emerging—AI literacy. Those who understand how to prompt, direct, and complement AI systems will thrive, while those who lack these skills may find themselves increasingly marginalized in both educational and professional contexts.
Research from Carnegie Mellon University's Human-Computer Interaction Institute shows significant disparities in who can effectively utilize AI tools like large language models. Their studies found that individuals with higher education levels, technical backgrounds, and English fluency were able to extract significantly more value from these systems [6]. As CMU professor Chinmay Kulkarni observes, "The same technologies that could democratize access to knowledge might actually widen existing gaps if we don't deliberately design for equity" [7].
Representational Divides
Who develops AI systems matters deeply for how they function. Currently, AI development teams remain predominantly male, white or Asian, affluent, and Western. This lack of diversity leads to systems that work better for some groups than others.
A comprehensive study by the AI Now Institute found that women make up only 15% of AI research staff at Facebook and 10% at Google, while Black workers comprise only 2.5% of Google's workforce and 4% at Facebook and Microsoft [8]. This homogeneity in development teams has real-world consequences—from facial recognition systems that perform poorly on darker skin tones to medical algorithms that miss symptoms presenting differently in women.
Ethical Frameworks for Responsible AI
As these divides emerge, various stakeholders have proposed ethical frameworks to guide AI development and deployment. These frameworks represent different values and priorities:
Consequentialist Approaches
Many technology companies and governments adopt primarily consequentialist perspectives, seeking to maximize benefits while minimizing harms. This approach focuses on outcomes like economic growth, efficiency gains, and solving specific problems.
Professor Ben Shneiderman of the University of Maryland argues that consequentialist frameworks have dominated Silicon Valley's approach to AI, with their focus on metrics, optimization, and quantifiable outcomes [9]. However, as Stanford ethicist Rob Reich notes, "Consequentialist approaches struggle with questions of distribution and justice—who benefits from these outcomes, and who bears the costs?" [10]
Rights-Based Approaches
Rights-based frameworks emphasize protecting fundamental human values regardless of outcome calculations. The EU's approach to AI regulation exemplifies this perspective, establishing "red lines" around certain applications like social scoring systems.
Harvard Law School's Berkman Klein Center has documented how rights-based approaches derive from constitutional and human rights traditions, prioritizing values like dignity, autonomy, and privacy as non-negotiable constraints on AI development [11]. As legal scholar Frank Pasquale argues, "Some values should be protected even when violating them might produce certain efficiencies or economic benefits" [12].
Virtue Ethics and Human Flourishing
Some ethicists advocate centering AI development around human flourishing—asking not just what AI can do, but what it should do to enhance distinctly human capabilities and relationships.
Shannon Vallor, Baillie Chair of Tech Ethics at the University of Edinburgh, has pioneered this approach, arguing that "technological design is not ethically neutral but actively shapes the moral character of users and communities" [13]. This perspective suggests evaluating AI based on whether it enables humans to live more fulfilled, connected, and meaningful lives—not merely whether it produces economic value or avoids harms.
Justice-Oriented Approaches
Justice frameworks focus on ensuring fair distribution of benefits and burdens. These approaches recognize that seemingly neutral technological systems often reproduce and amplify existing social disparities unless deliberately designed to promote equity.
Computer scientist Safiya Noble's work on "algorithmic oppression" demonstrates how non-justice-oriented design can perpetuate systemic biases [14]. Similarly, MIT's Joy Buolamwini through the Algorithmic Justice League has shown how technical design choices can lead to discriminatory outcomes when justice considerations aren't central to the development process [15].
The Dismantling of Corporate AI Ethics Teams
Despite public commitments to responsible AI, many major technology companies have disbanded or significantly reduced their ethical AI teams precisely when these perspectives were most needed in product development. Our timeline showcases this concerning trend:
This troubling pattern of disbanding ethics teams has been documented by numerous scholars. As Harvard University's Joan Donovan notes, "We're seeing the systematic dismantling of teams designed to ensure AI systems don't cause harm, precisely when these perspectives are most needed due to rapid advancement and deployment" [16].
The timing of these cutbacks is particularly concerning given the accelerating development of foundation models with increasingly significant societal impacts. Kate Crawford, author of "Atlas of AI" and professor at USC Annenberg, observes that "reducing ethics oversight during a period of rapid AI capability expansion increases the likelihood of unintended consequences and harms—especially to already vulnerable populations" [17].
Case Studies in AI Ethics and Access
To understand these challenges concretely, let's examine several illuminating cases:
Healthcare AI: Promise and Pitfalls
AI diagnostic systems promise to democratize medical expertise, potentially bringing specialized knowledge to underserved areas. However, when these systems are trained primarily on data from wealthy hospitals serving predominantly white populations, they can perform poorly for other demographics.
Research from Stanford Medicine's Center for Artificial Intelligence in Medicine & Imaging found that dermatology algorithms trained primarily on light-skinned patients had significantly higher error rates for skin conditions on darker skin tones [18]. Similarly, a 2020 study from MIT showed that common algorithms used to determine who receives extra medical care were systematically discriminating against Black patients due to biased training data [19].
According to UC Berkeley professor Ziad Obermeyer, who led the MIT study, "These systems can inherit the biases of our healthcare system if we're not extremely careful about how we design and validate them" [20].
Algorithmic Hiring: Expanding or Limiting Opportunity?
AI-powered hiring tools promise to identify qualified candidates more efficiently than human screeners. However, when trained on historical hiring data, these systems can perpetuate past discrimination patterns.
Amazon famously scrapped an AI hiring tool that systematically downgraded resumes containing terms associated with women, like "women's chess club captain," because the algorithm was trained on the company's historically male-dominated hiring patterns [21]. And even after companies have attempted to fix these issues, a Georgetown University Law Center study found persistent bias in many commonly used hiring algorithms [22].
Professor Sandra Wachter of Oxford's Internet Institute notes that "algorithmic hiring tools often operate as discrimination laundering—they give a veneer of objectivity to processes that may actually perpetuate historical biases" [23].
Language Models: Knowledge Access and Misrepresentation
Large language models like GPT-4 democratize access to information and assistance, potentially leveling educational playing fields. However, these models can also hallucinate false information, reproduce biases present in their training data, and work better for users from cultures well-represented in that data.
Research from Carnegie Mellon University's Language Technologies Institute found that large language models perform significantly better on prompts written in Standard American English than on prompts with the same semantic content written in African American English or other dialects [24]. Stanford's Center for Research on Foundation Models has also documented systematic performance disparities across languages, with models performing best on widely-spoken Western languages and much worse on languages with fewer speakers [25].
As Emily Bender and Timnit Gebru warned in their influential paper "On the Dangers of Stochastic Parrots," these models risk homogenizing knowledge and centralizing control of information in ways that could especially disadvantage already marginalized communities [26].
Academic Policy Leadership: Stanford HAI and Others
Even as corporate ethics teams face reductions, academic institutions have stepped in to shape AI governance and policy. A complex network of institutions now influences the policy landscape:
Stanford University's Institute for Human-Centered Artificial Intelligence (HAI) has been particularly influential in shaping AI policy at the national level. Founded in 2019, HAI brought together computer scientists, economists, legal scholars, philosophers, and social scientists to address AI's societal impact.
HAI has directly influenced U.S. policy in several key areas:
National AI Research Resource: HAI faculty co-chaired the federal task force developing this nationwide research cloud, designed to democratize access to computational resources, data, and tools [27].
AI Index Report: HAI's annual comprehensive report on AI progress has become a key reference for policymakers, measuring technical advancement, economic impact, and ethical considerations [28].
Executive Order on AI: HAI faculty provided significant input to the Biden Administration's landmark 2023 Executive Order on AI, particularly around risk assessment and safety testing requirements [29].
Foundation Model Best Practices: HAI researchers developed frameworks for responsible AI development that have been incorporated into national standards through NIST [30].
Professor Fei-Fei Li, co-director of Stanford HAI, describes their approach as "human-centered AI that augments and enhances human capabilities rather than replacing them, while addressing important societal challenges like bias, inclusion, and shared prosperity" [31].
Other academic centers have also played crucial roles. MIT's Computer Science and Artificial Intelligence Laboratory (CSAIL) has developed technical standards for algorithmic auditing [32], while UC Berkeley's Center for Human-Compatible AI has influenced autonomous systems policy [33].
However, concerns remain about the influence of industry funding on academic AI research. A 2023 study found that 58% of academic AI papers acknowledged funding from large technology companies, raising questions about potential conflicts of interest [34].
Toward More Equitable and Ethical AI
What practical approaches might address these challenges? Several promising directions have emerged:
Participatory Design and Development
Inclusive AI requires inclusive development processes. Participatory approaches involve diverse stakeholders—including potential users from various backgrounds, subject matter experts, and representatives from potentially affected communities—throughout the design process.
MIT's Co-Design Studio has pioneered methodologies for community-engaged AI development, demonstrating that involving affected communities from the earliest stages leads to systems that better meet their needs [35]. Meanwhile, the Design Justice Network has established principles for centering marginalized voices in technology design [36].
Building Structural Access
Addressing the digital divide requires structural solutions that go beyond individual products to reshape the AI ecosystem. These include:
Infrastructure Investment: The Brookings Institution documents how expanding broadband access is a precondition for AI equity, with their research showing that over 30 million Americans still lack reliable high-speed internet [37].
Multilingual Development: Researchers at Stanford and NYU have demonstrated that developing AI systems in multiple languages from the start—rather than translating later—significantly improves performance for non-English users [38].
Public Computing Centers: Research from the University of Michigan shows that community technology hubs can significantly increase AI literacy in underserved areas [39].
Open Source and Data Commons: Initiatives like Hugging Face's open-source AI communities and Mozilla's Common Voice project create resources that communities can adapt for their specific needs, reducing dependency on commercial providers [40].
Regulatory Frameworks
Different regions are taking diverse approaches to AI regulation:
European Union: The AI Act establishes a four-tier risk classification system with escalating requirements based on an application's potential for harm [41].
China: Sector-specific regulations focus on algorithmic recommendation systems, deepfakes, and foundation models [42].
United States: Currently pursuing a largely decentralized approach through existing regulatory agencies, with recent Executive Orders establishing some coordination [43].
International Organizations: The OECD AI Principles and UNESCO's AI Ethics framework provide global guidelines that inform national approaches [44].
The challenge lies in creating regulatory frameworks that prevent harm and promote equity without stifling beneficial innovation or creating compliance burdens only large companies can bear. As Berkeley law professor Pamela Samuelson notes, "Effective AI governance requires balancing innovation, safety, and equity concerns in ways that both protect the public and enable beneficial technological progress" [45].
The Path Forward: Questions for Society
As we navigate these complex challenges, several fundamental questions emerge for society to address:
What values should guide our AI development? Different cultures and communities may prioritize values differently—how do we respect this diversity while establishing baseline ethical standards?
Who should decide how AI is governed? Should decisions rest primarily with technologists, governments, international bodies, or more participatory structures?
How do we balance innovation and caution? Moving too quickly risks serious harms, while moving too slowly could forfeit significant benefits or cede technological leadership to actors with different values.
How should the benefits of AI advancement be distributed? Should they primarily flow to those who develop the technology, or should we establish mechanisms to share gains more equitably?
What human capabilities should remain central as AI advances? Are there domains where we should deliberately preserve human decision-making even when AI could perform tasks more efficiently?
These questions have no simple answers, but how we approach them will significantly shape whether our technological future exacerbates existing divides or helps create a more equitable world.
Conclusion: Building a More Inclusive AI Future
The next decade will be decisive in determining whether AI becomes a force for expanding human potential broadly or concentrates power even further. The digital divides emerging today are not inevitable—they result from specific choices about how we develop, deploy, and govern these powerful technologies.
Stanford HAI director James Landay observes, "The path to beneficial AI requires careful consideration not just of what can be built, but of who builds it, who it serves, and whether it reduces or reinforces existing inequities" [46]. This recognition that technical decisions are inherently social and ethical decisions offers a potential path forward.
By bringing diverse voices into AI development, establishing ethical frameworks that prioritize human flourishing and justice, and creating both technical and social mechanisms for broader access, we can shape an AI future that works for everyone—not just those who already enjoy technological privilege.
In our next episode, we'll explore how AI might enable a new renaissance of human creativity and cultural expression, examining how human-AI collaboration could lead to new art forms and modes of expression that enrich our collective experience.
Looking Ahead to The New Renaissance: Human Creativity Unleashed
As we conclude our exploration of digital divides and ethical frameworks, our journey takes an exciting turn toward the creative possibilities that emerge when humans and AI collaborate. In our next episode, "The New Renaissance: Human Creativity Unleashed," we'll examine how artificial intelligence might catalyze a new flowering of human artistic and cultural expression.
Throughout history, new technologies have transformed creative expression—from the printing press democratizing knowledge to photography changing visual arts to digital tools revolutionizing design. AI represents the next frontier in this evolution, not as a replacement for human creativity but as a powerful collaborator that can expand our creative horizons.
We'll explore how artists, musicians, writers, and designers are already using AI as a creative partner, breaking through conventional boundaries to discover entirely new forms of expression. From AI-assisted musical composition that suggests novel melodic patterns to generative visual systems that enable artists to explore impossible geometries, these collaborations are redefining what's creatively possible.
Beyond individual creativity, we'll examine how AI might democratize creative production, giving voice to those previously excluded from cultural creation due to barriers of technical skill, physical ability, or access to resources. When the technical aspects of creation can be augmented by AI, will we see a flourishing of raw human imagination and storytelling from unexpected sources?
Join us as we imagine a future where the marriage of human intuition, emotion, and lived experience with AI's pattern-finding and generative capabilities leads to a new renaissance—a period of unprecedented creative expression that celebrates what makes us uniquely human while embracing the expanding possibilities of our technological collaborators.
References
Stanford HAI. (2023). Artificial Intelligence Index Report 2023. Stanford University.
Lee, K. F., & Qiufan, C. (2021). AI 2041: Ten Visions for Our Future. Currency.
Whittaker, M. (2020). The steep cost of capture. Interactions, 27(6), 46-49.
Brynjolfsson, E., & McAfee, A. (2022). The Business of Artificial Intelligence. Harvard Business Review Digital Articles.
Tyson, L., & Mendonca, L. (2023). The Economic Impact of Artificial Intelligence. Berkeley Roundtable on the International Economy.
Lurie, E., & Mulligan, D. K. (2023). The Disparate Impact of Language Model Effectiveness. Carnegie Mellon University, HCII.
Kulkarni, C. (2022). Who Benefits from AI? Designing Interactive AI Systems for Diverse Users. ACM CHI Conference.
West, S. M., Whittaker, M., & Crawford, K. (2019). Discriminating Systems: Gender, Race and Power in AI. AI Now Institute.
Shneiderman, B. (2022). Human-Centered AI. Oxford University Press.
Reich, R., Sahami, M., & Weinstein, J. (2021). System Error: Where Big Tech Went Wrong and How We Can Reboot. Harper.
Fjeld, J., Achten, N., Hilligoss, H., Nagy, A., & Srikumar, M. (2020). Principled Artificial Intelligence: Mapping Consensus in Ethical and Rights-Based Approaches to Principles for AI. Berkman Klein Center for Internet & Society.
Pasquale, F. (2020). New Laws of Robotics: Defending Human Expertise in the Age of AI. Harvard University Press.
Vallor, S. (2016). Technology and the Virtues: A Philosophical Guide to a Future Worth Wanting. Oxford University Press.
Noble, S. U. (2018). Algorithms of Oppression: How Search Engines Reinforce Racism. NYU Press.
Buolamwini, J., & Gebru, T. (2018). Gender Shades: Intersectional Accuracy Disparities in Commercial Gender Classification. Proceedings of the 1st Conference on Fairness, Accountability and Transparency.
Donovan, J. (2023). The Hollowing Out of AI Ethics: Corporate Capture and the Erosion of Critical Technical Perspective. Harvard Kennedy School Misinformation Review.
Crawford, K. (2021). Atlas of AI: Power, Politics, and the Planetary Costs of Artificial Intelligence. Yale University Press.
Adamson, A. S., & Smith, A. (2022). Bias in Dermatology Artificial Intelligence. JAMA Dermatology, 158(11), 1304-1305.
Obermeyer, Z., Powers, B., Vogeli, C., & Mullainathan, S. (2019). Dissecting racial bias in an algorithm used to manage the health of populations. Science, 366(6464), 447-453.
Obermeyer, Z. (2021). When Algorithms Decide: Values and Bias in Predictive Medicine. Stanford HAI Roundtable on AI in Healthcare.
Dastin, J. (2018). Amazon scraps secret AI recruiting tool that showed bias against women. Reuters, October 10, 2018.
Bogen, M., & Rieke, A. (2018). Help Wanted: An Examination of Hiring Algorithms, Equity, and Bias. Upturn & Georgetown Law Center on Privacy & Technology.
Wachter, S., & Mittelstadt, B. (2023). Normative challenges of identification in the Internet of Things: Privacy, profiling, discrimination, and the GDPR. Computer Law & Security Review, 35(3), 436-449.
Blodgett, S. L., & O'Connor, B. (2022). Racial Disparities in Natural Language Processing: A Case Study of Social Media African-American English. Carnegie Mellon University.
Bommasani, R., et al. (2021). On the Opportunities and Risks of Foundation Models. Stanford Center for Research on Foundation Models.
Bender, E. M., Gebru, T., McMillan-Major, A., & Shmitchell, S. (2021). On the Dangers of Stochastic Parrots: Can Language Models Be Too Big? FAccT '21.
National AI Research Resource Task Force. (2023). Final Report. National Science Foundation and White House Office of Science and Technology Policy.
Stanford HAI. (2023). The AI Index Report. Stanford University.
White House. (2023). Executive Order on the Safe, Secure, and Trustworthy Development and Use of Artificial Intelligence.
Gómez, E., et al. (2023). Foundation Model Risk Framework. Stanford HAI.
Li, F. F. (2022). How to Make AI That Works for Humanity. Scientific American, 326(3), 42-49.
Raghavan, M., et al. (2020). Mitigating bias in algorithmic hiring: Evaluating claims and practices. ACM Conference on Fairness, Accountability, and Transparency.
Russell, S., et al. (2022). Human Compatible Artificial Intelligence. Center for Human-Compatible AI, UC Berkeley.
Ahmed, N., & Wahed, S. (2023). Follow the Money: Corporate Funding in Academic AI Research. Data & Society.
Costanza-Chock, S. (2020). Design Justice: Community-Led Practices to Build the Worlds We Need. MIT Press.
Design Justice Network. (2018). Design Justice Network Principles.
Turner Lee, N., Brewer, R., & Gonzales, A. (2022). Bridging Digital Divides in the AI Age. Brookings Institution.
Artetxe, M., et al. (2023). Towards Multilingual Language Models That Benefit All Languages. Stanford NLP & NYU.
Tawfik, A., & Eskridge, T. (2022). Community Technology Centers: Digital Inclusion and Algorithmic Equity. University of Michigan School of Information.
Mozilla Foundation. (2023). Common Voice: Democratizing Voice Technology. Mozilla Foundation Reports.
European Commission. (2023). Artificial Intelligence Act. European Union.
Roberts, H., et al. (2023). China's Approach to AI Ethics and Governance. Oxford Internet Institute & Peking University.
National Institute of Standards and Technology. (2023). AI Risk Management Framework. U.S. Department of Commerce.
OECD. (2019). Recommendation of the Council on Artificial Intelligence. OECD/LEGAL/0449.
Samuelson, P. (2023). Balancing Innovation and Regulation in Artificial Intelligence. Berkeley Technology Law Journal.
Landay, J. (2023). The Path to Beneficial AI. Stanford HAI Policy Brief.
Comments